Data Science and Data Engineering are two of the most important disciplines in today’s data-driven world. They both involve collecting, storing, analyzing, and interpreting large amounts of data in order to make better decisions and understand how a company operates. However, these two fields differ greatly in their approach and skill set.
This blog post will explore the differences between Data Science and Data Engineering as well as how they complement each other.
By understanding the different roles that each field plays, businesses can get the most out of their data assets while maintaining a high level of accuracy.
So lets dive into what exactly makes Data Science and Data Engineering unique!
What is Data Science?
Data Science is a field that utilizes scientific methods, processes, algorithms and systems to extract knowledge and insights from structured and unstructured data.
Data Scientists use mathematical models, computer science programs and statistical techniques to analyze data sets in order to identify patterns or trends. This helps them make predictions about future events or outcomes, as well as uncover relationships between variables.
Data Scientists also collaborate with stakeholders in order to develop solutions for business problems. They often work on projects such as predictive analytics, machine learning, natural language processing (NLP), data visualization, and deep learning.

The ultimate goal of Data Science is to help organizations gain meaningful insights from their big data in order to improve decision-making processes.
The field of Data Science is often confused with another significant data management field and that is Data Analytics. If you wish to know more about this topic, read our blog on Data Science vs Data Analytics
Acquire expertise in consonance with that of a Data Analyst, enroll today for the Data Analytics course at Syntax Technologies.
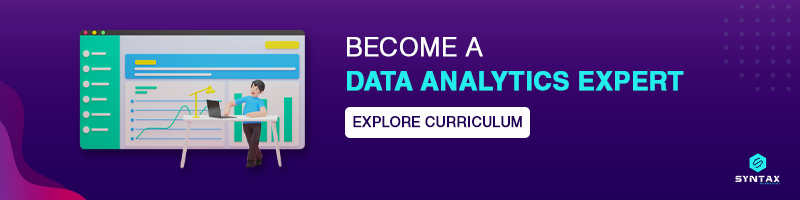
What is Data Engineering?
Data Engineering is the process of building, maintaining, and optimizing data processing systems. It involves collecting, storing, cleaning, transforming and preparing data for analysis.
Data Engineers often design architectures such as databases and data warehouses that allow companies to analyze their data in the most efficient way possible.
They also develop data mining pipelines for transferring and streaming data from multiple sources as well as creating applications to manage large datasets. Additionally, they may create software tools to automate the collection and cleanup of messy datasets.
The ultimate goal of Data Engineering is to ensure that data processing systems are reliable, efficient and scalable. It enables companies to make better decisions by taking advantage of the vast amounts of available data.
Read more about the field of Data Engineering in detail, refer to our blog on What is Data Engineering?
Data Science vs Data Engineering: Exploring the Differences between the Two
Data Science and Data Engineering are two interconnected fields that play a vital role in extracting meaningful insights from data. While Data Science focuses on analyzing and interpreting data, Data Engineering lays the foundation for data collection and processing.
Let’s embark on a journey to explore their similarities, differences, and determine which field takes the lead in various aspects.
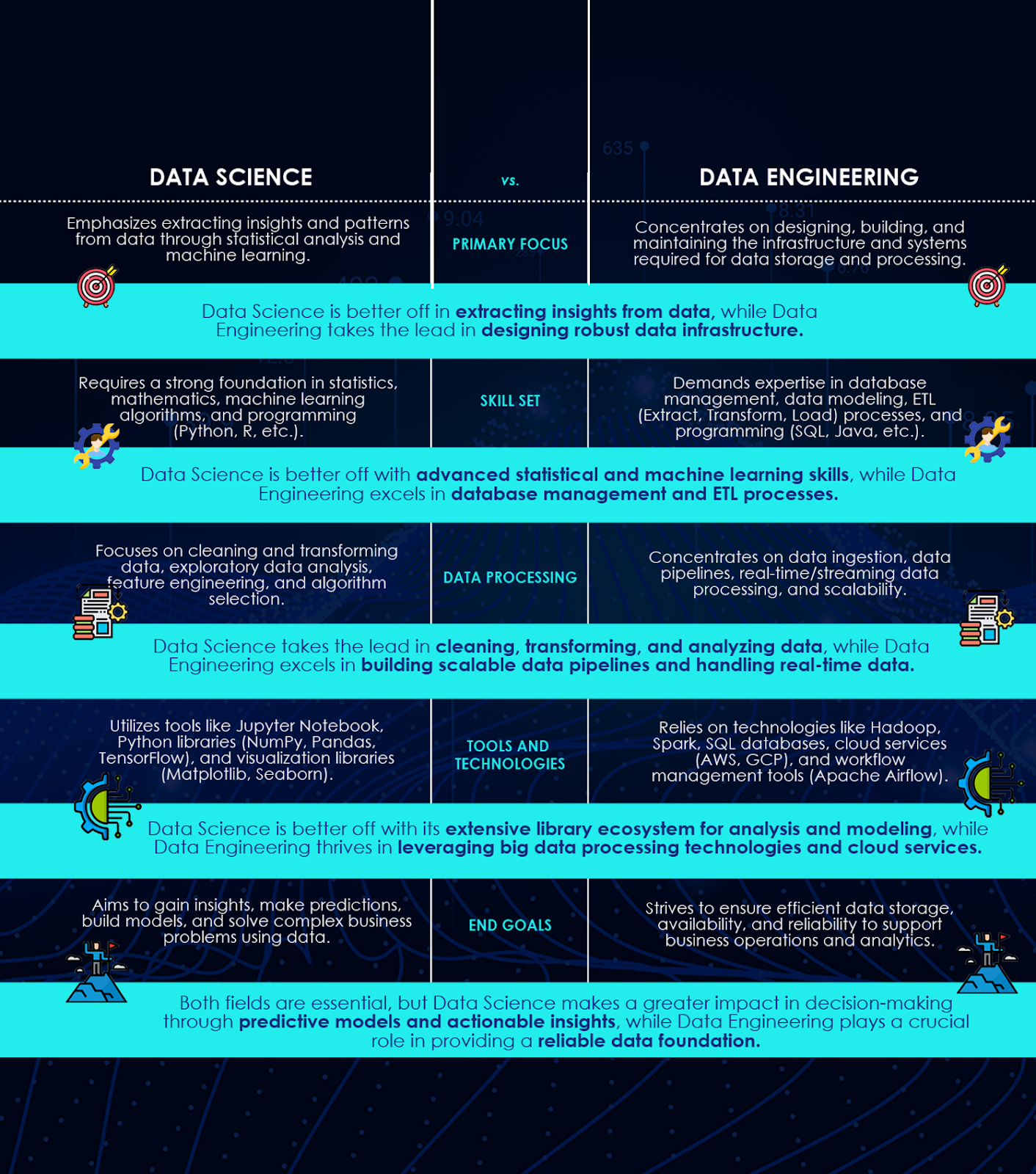
Pros and Cons of a Career in either field
Pros and Cons of a Career in Data Science:
Pros:
- High demand: Data scientists are in high demand across industries due to the need for insights from data.
- Lucrative salaries: Data scientists receive competitive salaries and attractive compensation packages.
- Intellectual stimulation: Data science involves solving complex problems and continuous learning.
- Impactful work: Data professionals make a significant impact through data-driven insights and decision-making.
- Versatile applications: Data science is applicable in various fields, providing flexibility and specialization opportunities.
Cons:
- Steep learning curve: Data science requires a strong foundation in mathematics, programming, and machine learning.
- Evolving field: Data science is constantly evolving, requiring professionals to stay updated.
- Data quality issues: Real-world data can have quality issues that affect analyses.
- Ambiguity in problem statement: Projects may lack clear objectives, requiring navigation through ambiguity.
- Ethical considerations: Data scientists must consider privacy, bias, and fairness when working with data.
Pros and Cons of a Career in Data Engineering:
Pros:
- Growing demand: Data engineering professionals are in high demand to build robust data infrastructures.
- Stable career path: Data engineering offers stability as organizations rely on data for decision-making.
- Technical expertise: Data engineering involves cutting-edge technologies, fostering strong technical skills.
- Variety of challenges: Data engineering offers diverse and interesting challenges.
- Collaborative work: Data engineering involves collaboration with cross-functional teams.
Cons:
- Complex infrastructure: Building and managing data infrastructures require expertise in various technologies.
- Constant learning: Data engineers and data scientists must stay updated with evolving technologies and best practices.
- Data quality and integrity: Ensuring data quality is crucial and requires attention.
- Limited visibility: Data engineering work may have less immediate visibility compared to other roles.
- Infrastructure maintenance: Data engineering involves ongoing maintenance and optimization of data pipelines.
Identifying opportunities for Overlap between the two fields
Data Science and Data Engineering have complementary skill sets that can be used to build powerful and innovative solutions.
For example, a data engineer may use their expertise in database design to create a structure that maximizes data analysis capabilities. In turn, a data scientist can leverage their insights to make predictions about future trends or outcomes.
The best results are achieved when both disciplines work together in harmony. It is important for companies to identify potential areas of overlap and develop processes that allow the two teams to collaborate effectively. This will ensure that they can quickly and efficiently gain meaningful insights from their data assets.
Conclusion
Data Science and Data Engineering are two important fields that together form a powerful data-driven workflow. Data Scientists use mathematical models, computer programs and statistical techniques to analyze data sets in order to identify patterns or trends, while Data Engineers build, maintain, and optimize raw data processing systems.
By recognizing the similarities between the two fields and identifying areas of overlap, companies can develop efficient processes for gaining meaningful insights from their data assets. The best results come when both disciplines work together in harmony, allowing organizations to make more informed decisions based on reliable data analysis.